The Community Development team works to understand the economic experiences of lower-income households and communities to help build a stronger economy for all Americans.
We conduct research on challenges and promising practices related to economic opportunity.
We conduct and share data-driven research that tells a nuanced story.
We cultivate and strengthen multisector partnerships to expand economic opportunity.
We facilitate dialogue, shared learning, and partnership building across sectors. We hold events, roundtables, and one-on-one conversations to surface promising strategies. We help connect communities to new resources, new partners, and new ideas.
We engage our communities to better understand the Twelfth District.
By connecting with our stakeholders, we learn about current challenges and emerging issues and elevate them to inform Bank leadership. We dialogue with a broad range of contacts to gather information that can inform monetary policy.
Receive updates from our team
Our Research
Research publications on community development trends and issues.
In-depth analysis of emerging community development issues from practitioners and scholars.
Regional data profiles and analysis on community development-related issues.
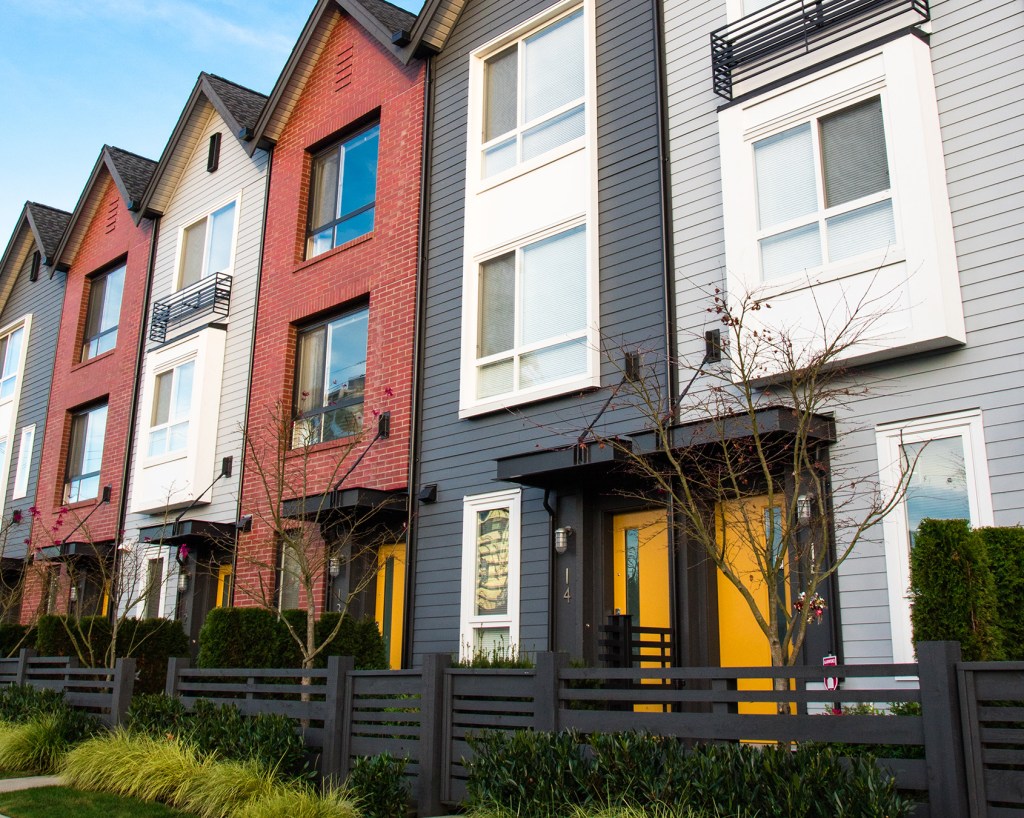
Homeownership Opportunities Beyond Single-Family: Quantifying the Current Landscape
Although homeownership is commonly portrayed as a single household living in a detached house, homeownership in the U.S. includes an array of opportunities, some of which provide more affordable entry points into owning a home.
The Shifting Landscape of Job Proximity: A Conversation with Visiting Scholar Scott Allard
SF Fed Visiting Scholar Scott Allard and Assistant Vice President of Community Development Research Elizabeth Kneebone discuss the importance of job proximity, especially for lower-income workers, and their research collaboration focused on understanding how proximity to jobs has shifted for low-income workers and communities since the pandemic.
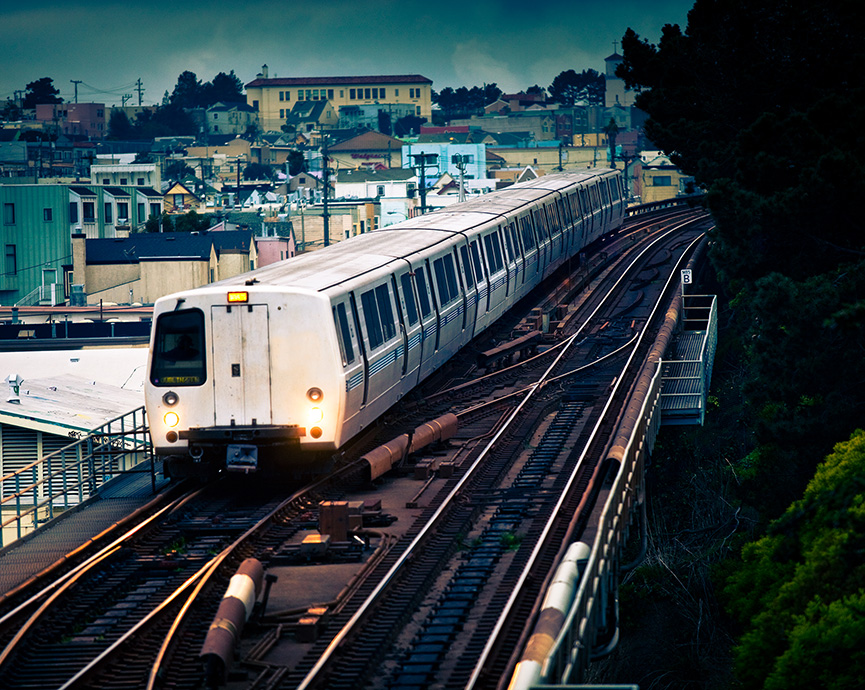
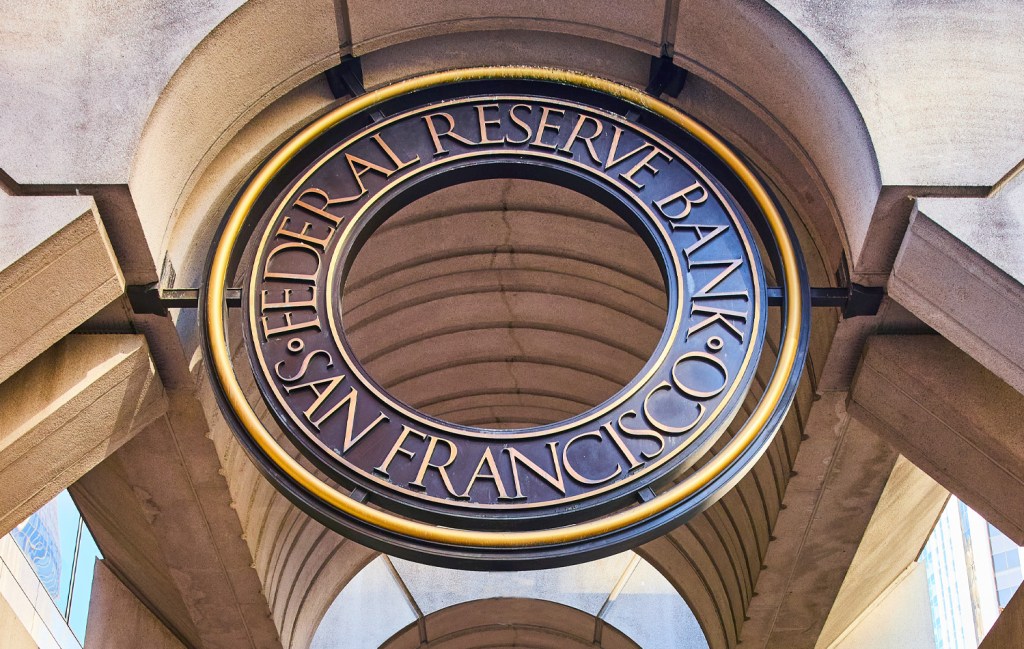
Visiting Scholars in
Community Development
Visiting Scholars engage with department staff to share experiences and best practices from the field, advise current and proposed initiatives, and share their research with community development stakeholders.